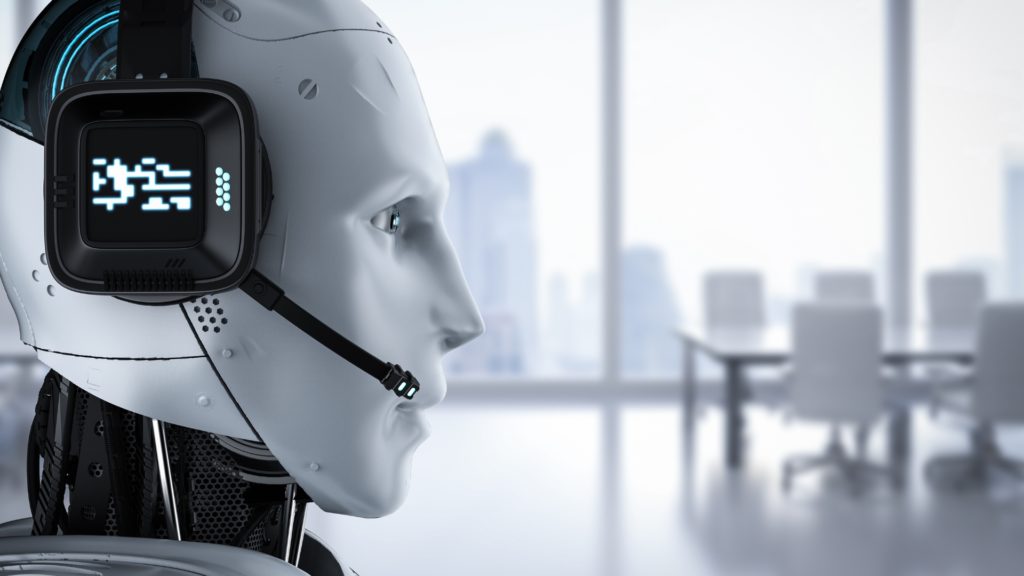
In the information technology (IT) ecosystem, the integration of artificial intelligence (AI) and machine learning (ML) is radically transforming business operations, providing significant advancements in efficiency and innovation. As the deployment of AI-based solutions in business processes accelerates, the implementation of robust security measures becomes crucial. This article explores essential practices for ensuring secure integration of AI, highlighting the crucial role of the MLSecOps approach and presenting indispensable tools to navigate this complex landscape.
Ensuring Secure Integration of AI in IT Enterprises.
The adoption of AI and ML technologies promises to enhance data-driven decision-making, automate repetitive tasks, and optimize business processes. However, this integration poses unique challenges in terms of data security and information system protection. Adopting an AI-centered cybersecurity strategy is essential to prevent data breaches and secure critical infrastructures.
The challenges of adopting AI
The adoption of AI and ML introduces new risks, including an expanded attack surface, algorithmic biases, privacy and copyright violations, as well as IT infrastructure risks . It is crucial to establish robust security strategies to protect corporate data and systems.
Overcoming Security Challenges with MLSecOps
MLSecOps, a methodology that integrates best security practices into the lifecycle of AI and ML projects, proves to be an effective response to security concerns. By infusing security considerations from the early stages of development and throughout the operationalization of ML models, companies can better protect their digital assets against emerging threats.
MLSecOps: A Framework for AI Security
MLSecOps represents a natural evolution of MLOps, integrating security practices into the lifecycle of ML models. This approach aims to secure both the data used to train and test ML models, as well as the IT infrastructure used to host and run them.
The pillars of MLSecOps security
The main security strategies under the MLSecOps approach include supply chain vulnerability management, model provenance verification, governance, risk, and compliance (GRC), trusted AI, and adversarial machine learning. These strategies aim to mitigate risks and ensure that ML models are developed, tested, deployed, and monitored using best security practices.
The implementation of MLSecOps
The implementation of MLSecOps requires a pragmatic approach, starting with data integration and analysis, the development of tailored ML models, the orchestration of automated responses, and ongoing monitoring to enhance incident response strategies. MLSecOps enables accelerated incident detection, minimizes response times, reduces human errors, and enhances incident management.
Tools and Technologies for Secure AI Integration
The choice of AI tools and platforms plays a crucial role in securing AI integration. Solutions like AI as a Service (AIaaS) platforms provide secure environments for developing and deploying AI applications while ensuring compliance with security and data protection standards.
Recommended tools :
- GenAI Builder from SnapLogic : This tool facilitates the integration of internal company data with AI models, using the Retrieval Augmented Generation (RAG) approach to teach AI models specific company data. It is compatible with a wide range of data and can be used for customer support automation, data analysis, contract review, and marketing personalization .
Generative AI and GenAI Builder: A Revolution in the IT Sector
The SnapLogic GenAI Builder is an innovative example of a tool that allows IT sector businesses to create secure and customized applications using large language models (LLM). This platform automates enterprise data integration, generating new insights and business processes while improving the relevance and accuracy of AI responses.
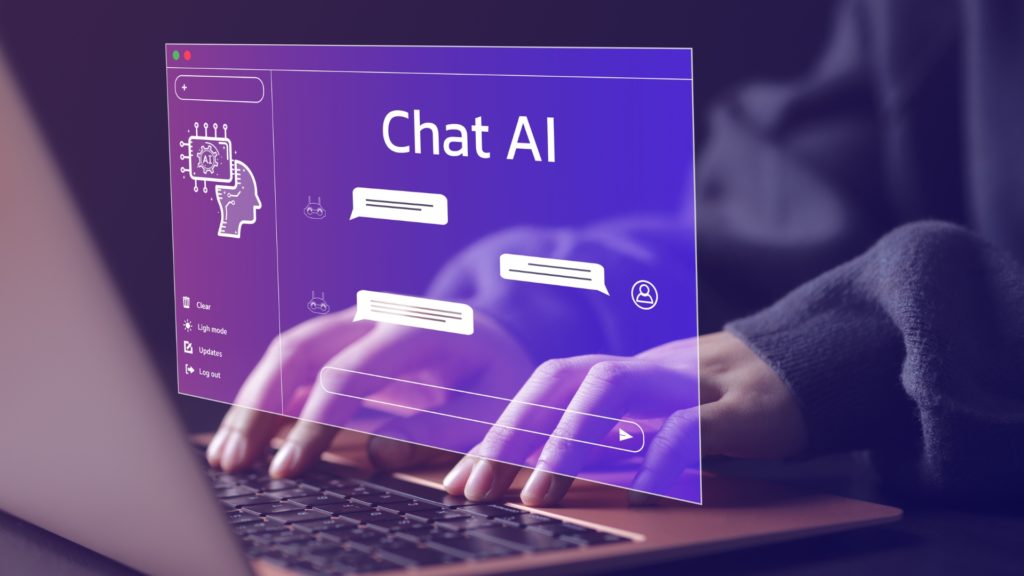
The integration of AI and ML represents a new frontier for businesses in the field of IT, promising unprecedented efficiency gains and innovation. By adopting an MLSecOps approach and carefully selecting secure tools, companies can address the security challenges associated with AI, ensuring a successful and secure integration. The IT landscape continues to evolve with AI, and staying at the forefront of security is essential to fully harness the potential of these disruptive technologies.
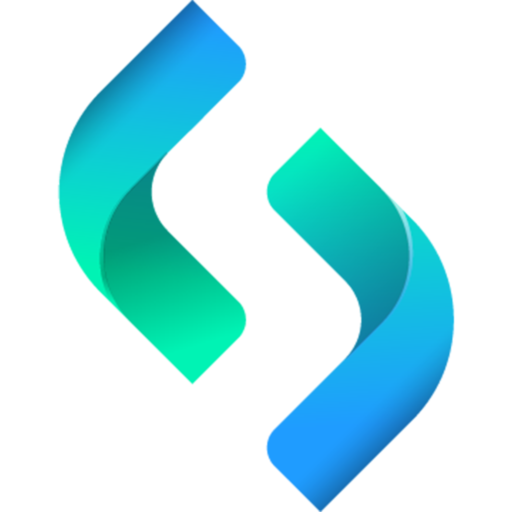